Big Spatial Data covers a wide spectrum of data types including (a) Raster data, e.g. Geoimages, (b) Vector data, e.g., Points, Lines, Polygons, and (c) Graph data, e.g. Road network graph. Thus, “Big Spatial Data” deals with massive amounts of real-time spatial and spatio-temporal data obtained from billions of sensors, location-aware devices, remoting sensing satellites, and various models of the physical world. The use of Big Spatial Data spans a variety of applications including social networks, earth sciences, transportation, communication networks, online maps, smart cities and urban planning, remote sensing, and crisis and evacuation management, to name but a few. Turning Big Spatial Data into value is challenging and requires introduction of fundamentally new spatio-temporal algorithms, methods, and systems that can process, mine, and analyze massive amounts of fast and heterogeneous spatiotemporal data in a timely manner.
The aim of this workshop is to bring together researchers from academia, government and industry who are actively addressing problems in the area of Big Spatial Data management and analysis. This workshop offers the audience the opportunity to discuss the lessons which they have learned over the years, to demonstrate what they have achieved so far, and to plan for the future of “Big Spatial Data”.
Call for Papers
We encourage researchers from academia and industry to submit papers that highlight the value of Big Spatial Data processing, management, mining, and analysis on topics that include, but are not limited to the following:
- ● Big Spatial Data: Fundamentals and Theory
- ● Big Spatial Data: Management
- ● Big Spatial Data: Mining and Analysis
- ● Big Spatial Data: Stream Processing
- ● Big Spatial Data: Spatial Time Series Querying and Mining
- ● Big Spatial Data: Spatial Graph Processing
- ● Big Spatial Data: Descriptive, Predictive, and Prescriptive Models
- ● Big Spatial Data: Privacy and Authentication
- ● Big Spatial Data: Geosensing
- ● Big Spatial Data: Indexing
- ● Big Spatial Data: Modern Hardware, High Performance Computing, and Cloud
- ● Big Spatial Data: Visualization
- ● Big Spatial Data: Deep Learning
- ● Big Spatial Data: NoSQL and NewSQL Data Stores
- ● Big Spatial Data: Data Frameworks
- ● Big Geospatial Information Retrieval and Crowdsourcing
- ● Big Geosocial Networks
- ● Big Spatial Data Applications: Smart Cities and Intelligent Transportation, Urban Computing, Healthcare, Geosciences, etc.
Important Dates
Paper Submission Deadline: Oct 1, 2019 Oct 15, 2019
Notification of Acceptance: Nov 1, 2019
Camera-Ready Submissions: Nov 15, 2019
Workshop Date: December 9-12, 2019
Submission
Authors with interests in any of the listed topics or any other topic related to Big Spatial Data paradigm are cordially invited to submit their work. All submissions should be in high quality, original and not published or under review elsewhere during the review process.
Submitted papers have to follow the IEEE official template. Maximum paper length allowed is:
Full Papers: 10 pages
Short (work-in-progress) Papers: 4 pages
Demo Papers: 4 pages
Position/Vision Papers: 4 pages
If from last three categoizes listed above, the title of the paper should start with “Short Paper:”, “Demo Paper:”, or “Vision Paper:”, respectively. Submitted papers will be reviewed by members of the Workshop Program Committee. At least one author for each accepted paper has to register and present the work.
Paper submission website: click here!
Keynote: Harnessing the Power of Big Spatial Data with Privacy
Abstract: Big spatial data collected from increasingly prevalent sensors and users with location-aware devices bring unprecedented opportunities to data-driven intelligence. Yet the massive collection and usage of personal location and trajectory data by service providers and other third parties could lead to disclosure of sensitive information and raise significant privacy concerns. In this talk, I will review the differential privacy framework and present its techniques and applications in various spatial data sharing and usage settings including: 1) centralized setting (a trusted data collector or service provider shares aggregated data and models with other third parties), 2) local setting (an untrusted service provider collects data from individual users to support location based applications such as POI search and spatial crowdsourcing and at the same time enable aggregated data analytics), and 3) federated or collaborative setting (multiple service providers share the data for joint learning). I will highlight the unique challenges of spatial data due to its inherent spatiotemporal correlations and present studies addressing them in these contexts.
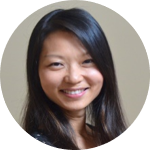
Bio: Li Xiong is a Professor of Computer Science and Biomedical Informatics at Emory University. She held a Winship Distinguished Research Professorship from 2015-2018. She has a Ph.D. from Georgia Institute of Technology, an MS from Johns Hopkins University, and a BS from the University of Science and Technology of China. She and her research lab, Assured Information Management and Sharing (AIMS), conduct research on algorithms and methods for big data management, data privacy and security, in the context of spatiotemporal and health data. She has published over 130 papers including six best paper (runner up) awards. She currently serves as associate editor for IEEE Transactions on Knowledge and Data Engineering (TKDE), program co-chair for ACM SIGSPATIAL 2018, 2020 and 2021, program vice-chair for IEEE International Conference on Data Engineering (ICDE) 2020, and on many program committees for data science and data security conferences. Her research is supported by National Science Foundation (NSF), AFOSR (Air Force Office of Scientific Research), National Institute of Health (NIH), and Patient-Centered Outcomes Research Institute (PCORI). She is also a recipient of Google Research Award, IBM Smarter Healthcare Faculty Innovation Award, Cisco Research Award, AT&T Research Gift, and Woodrow Wilson Career Enhancement Fellowship. She is an ACM distinguished scientist.
Program:
Start | End | Event |
---|---|---|
7:00 | 8:15 | Registration |
8:15 | 8:30 | Opening and Welcome |
8:30 | 10:15 | Paper Presentation Session: Data Management |
8:30 | 8:50 |
Large Scale Spatial Data Processing With User Defined Filters In BboxDB
|
8:50 | 9:10 |
Euler++: Improved Selectivity Estimation for Rectangular Spatial Records
|
9:10 | 9:30 |
bench4gis: Benchmarking Privacy-aware Geocoding with Open Big Data
|
9:30 | 9:50 |
Towards Building Evacuation Planning Platform using Multimodal Transportation for a Large Crowd: Work in progress
|
9:50 | 10:15 |
SEXTANT: A Computational Framework for Scalable and Efficient Correlation of Spatio-Temporal Trajectories
|
10:15 | 10:30 | Coffee Break (California Foyer) |
10:30 | 11:55 | Paper Presentation Session: Pattern Discovery |
10:30 | 10:50 |
Accurate Spatial Mapping of Social Media Data with Physical Locations
|
10:50 | 11:10 |
Co-location Pattern Mining of Geosocial Data to Characterize Urban Functional Spaces
|
11:10 | 11:35 |
Monitoring of natural disasters through anomaly detection on mobile phone data
|
11:35 | 11:55 |
User Identification across Online Social Networks Based on Similarities among Distributions of Friends' Locations
|
12:00 | 1:30 | Lunch (On Your Own) |
1:30 | 3:40 | Paper Presentation Session: Learning and Data Mining |
1:30 | 1:50 |
Evaluation of Location Estimation Method That Focuses on Geographical Proximity of Friends
|
1:50 | 2:15 |
Mining top-up transactions and online classified ads to predict urban neighborhoods socioeconomic status
|
2:15 | 2:35 |
Spatio-temporal classification at multiple resolutions using multi-view regularization
|
2:35 | 3:40 | Keynote: Li Xion (Emory University) |
3:40 | 4:00 | Coffee Break (California Foyer) |
4:00 | 5:30 | Paper Presentation Session: Data Analysis and Visualization |
4:00 | 4:25 |
Decision-Making System for Road-Recovery Considering Human Mobility by Applying Deep Q-Network
|
4:25 | 4:45 |
cPSITRES: A collabrative system for analysis of Big Data on sea ice
|
4:45 | 5:05 |
A demonstration of B-EagleV: Visualizing massive point cloud directly from HDFS
|
5:05 | 5:30 |
BigDataCube: A Scalable, Federated Service Platform for Copernicus
|
5:30 | 6:00 | Adjourn |
Organizers
Workshop Co-chairs
Farnoush Banaei-Kashani, farnoush.banaei-kashani@ucdenver.edu, University of Colorado Denver
Farnoush Banaei-Kashani is currently an assistant professor at the Department of Computer Science and Engineering, College of Engineering and Applied Science, University of Colorado Denver. Previously, he was a research scientist at the Computer Science Department, University of Southern California (USC), where he also earned his PhD degree in Computer Science and MS degree in Electrical Engineering in 2007 and 2002, respectively. Dr. Banaei-Kashani is passionate about performing fundamental research toward building practical, large-scale data-intensive systems, with particular interest in Data-driven Decision-making Systems (DDSs), i.e., systems that automate the process of decision-making based on (big) data. In the past he has introduced a number of novel DDSs in various application areas (including, transportation, health, safety and security, energy, and scientific computing). Dr. Banaei-Kashani has published more than 65 referred papers and has received several awards. His research has been supported by grants from both governmental agencies (NSF/CENS, NIH/CTSI, DOT/METRANS, DOJ/NIJ and NASA/JPL) and industry (Google, IBM, Chevron and NGC).
Siyuan Lu, lus@us.ibm.com, IBM
Siyuan Lu is currently a Manager and Principal Research Staff Member leading the Data Intensive Physical Analytics group at the IBM T. J. Watson Research Center, Yorktown Heights, NY. He received the Ph.D. degree in Physics from University of Southern California (USC) in 2006. Prior to joining IBM in 2012, he was an Assistant Research Professor jointly appointed in the Department of Physics and the Department of Ophthalmology at USC. Dr. Lu’s current research interests at IBM include architectures of geospatial data services and the union of physics and data-driven approaches for modeling complex systems with applications in renewable energy forecasting, climate forecasting, and environmental monitoring. He technically leads the Department of Energy funded project on solar energy forecasting and the development of IBM PAIRS geospatial Big Data services. He has co-authored over 50 peer-reviewed articles and has served as members on multiple governmental committees.
Chengyang Zhang, cyzhang@amazon.com, Amazon
is currently a Software Development Manager at Amazon.com leading a team to build end to end Machine Learning system for Amazon’s retail pricing systems. He completed his PhD in Computer Science from the University of North Texas in 2011. Dr. Zhang is actively working on various projects related to database query optimization and big data systems. His research interests include data warehousing, Internet of Things, big data systems, geo-streaming data processing, spatial databases and data mining. Dr. Zhang has published over 20 peer reviewed papers and owns two patents.
Abdeltawab Hendawi, hendawi@uri.edu, University of Rhode Island
Abdeltawab Hendawi is currently an Assistant Professor Computer Science at the University of Rhode Island. He is also the Co-Director of the AI at URI. He obtained his MSc and PhD in Computer Science and Engineering from the University of Minnesota, Twin Cities. His research interests are centered on the broad area of Data Science (big data management and analytics) with more focus on smart cities and smart health related applications. His work has been recognized by a number of awards, including the Best Paper Award at ACM SIGSPATIAL MobiGIS 2012; Best Design/Plan Poster Award and Best Overall Poster Award at the U-Spatial Symposium 2013; Best Demo Paper Award at ACM SIGSPATIAL 2014; Data Science Research Fellowship at the University of Washington 2014; Best and Second Best Demo Paper Awards at IEEE MDM 2015; Hobby Postdoc Research Fellowship at the University of Virginia 2015; Blue Sky Ideas Award 2016 and Best Runner-up Vision Paper Award at ACM SIGSPATIAL 2016; Best Poster Award at the UVa Research Symposium 2018; and Best Demo Paper Award Runner-up at ACM SIGSPATIAL 2018.
Program Committee
● Mahdi Boukhechba, University of Virginia
● Ahmed Eldawy, University of California Riverside
● Yan Huang, University of North Texas
● Mohamed Khalifa, Alexandria University
● Xiao Liu, University of Arkansas
● Amr Magdy, University of California, Riverside
● Dev Oliver, ESRI
● Ayman Taha, Technological University Dublin
● Fusheng Wang, Stony Brook University
● Jianting Zhang, City College of New York and CUNY Graduate Center
● Demetris Zeinalipour, University of Cyprus”
Webmaster
● Shahab Helmi, shahab.helmi@ucdenver.edu